Sprinkler Bayes Net. Suppose that a sprinkler (S) or rain (R) can make the grass in your yard wet (W). The probability that the sprinkler was on depends on whether the day was cloudy (C). The probability of rain also depends on whether the day was cloudy. The DAG for events C,S,R, and W is shown in Fig. 3.19.
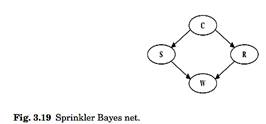
Diabetes in Pima Indians. The Pima Indians have the world’s highest reported incidence of diabetes. Since 1965, this population has participated in a longitudinal epidemiological study of diabetes and its complications. The examinations have included a medical history for diabetes and other major health problems. A population of women who were at least 21 years old, of Pima Indian heritage, and living near Phoenix, AZ was tested for diabetes according to World Health Organization criteria.
The following conditions (“events”), constructed from the database, can be related to a randomly selected subject from this population.
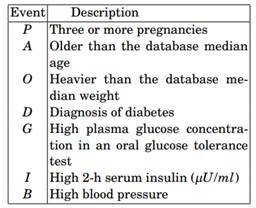
The DAG in Fig. 3.20 simplifies the proposal of Tom Bylander from the University of Texas in San Antonio, who used Bayesian networks and the Pima Indians Diabetes Database in a machine learning example.4
From 768 complete records relative frequencies are used to approximate the conditional probabilities of the nodes. The probabilities are given in the following tables.
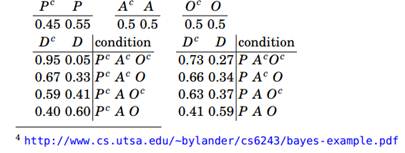